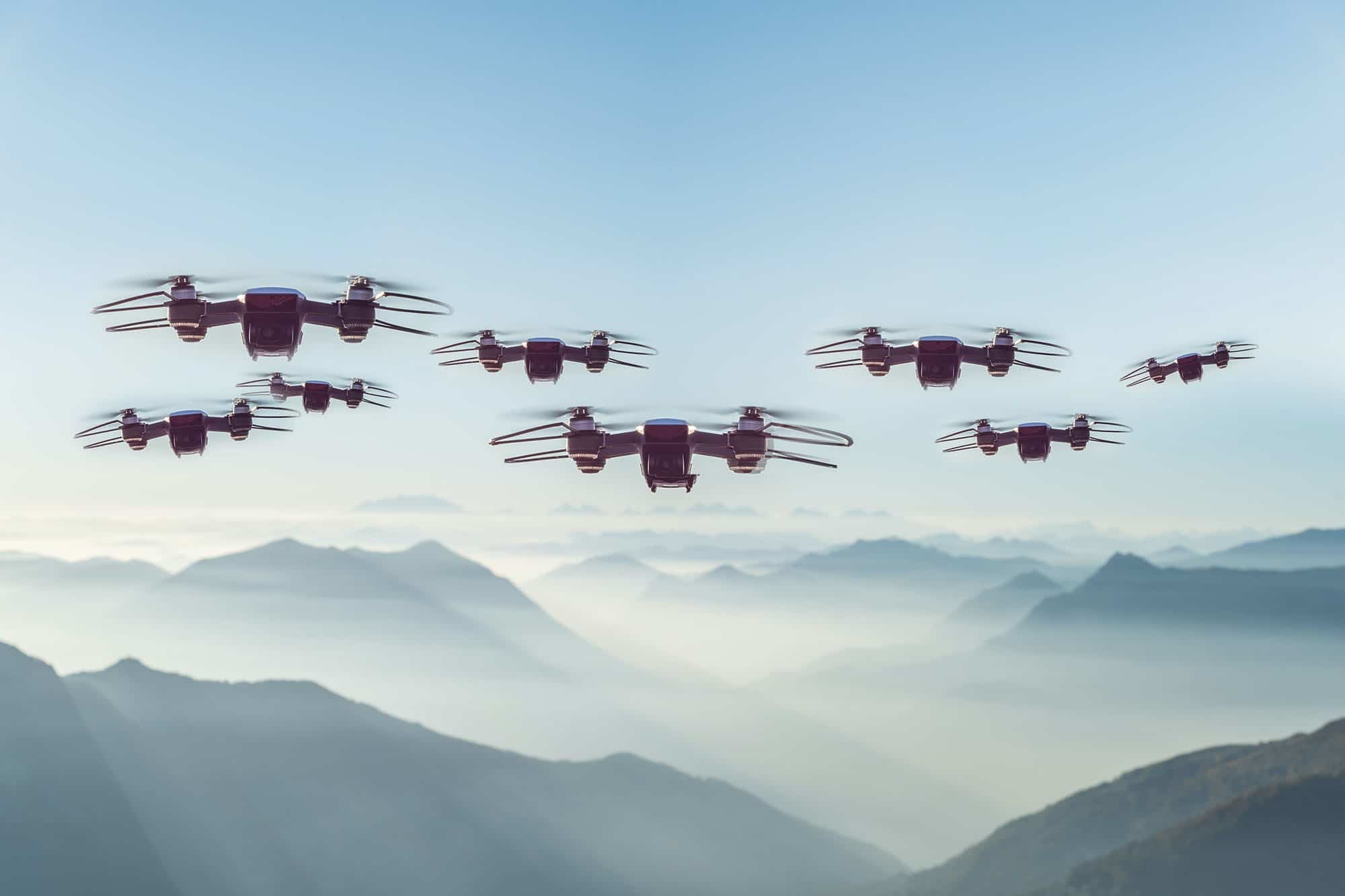
Research Advances Learning Capability of Drone Swarms
Researchers, including Aranya Chakrabortty, have developed a reinforcement learning approach that will allow swarms of unmanned vehicles to accomplish various missions while minimizing performance uncertainty.
August 31, 2020 Charles Hall
It is common for multi-agent systems in a battlefield to be restricted by strict deadlines for taking control actions and to lack the resources to install a large number of reliable or fast enough communication links. In such circumstances, solving a common target-tracking or motion planning control problem in real-time can be highly challenging, or even impossible. The problem becomes even more complex when the network model is unknown to the designer.
Aranya Chakrabortty , professor of electrical and computer engineering at North Carolina State University, and his research group in collaboration with researchers from the Army Research Lab (ARL) and Oklahoma State University have developed a new machine learning-based control strategy to resolve this problem. In their recent paper published in the American Control Conference 2020 (with an extended journal version available on Arxiv) they propose a hierarchical reinforcement learning (RL) based control scheme for extreme-scale multi-agent swarm networks where control actions are taken based on low-dimensional sensed data instead of models. The approach is to decompose a large-dimensional control objective into multiple smaller hierarchies – for example, multiple small group-level microscopic controls that can be learned using local group-level data only, and a broad system-level macroscopic control that steers the swarm in its desired direction but using only high-level sparse data.
One pertinent example is target-tracking using swarms of ground vehicles and air vehicles. The air vehicles serve as higher-level coordinators that provide a macroscopic view of the target motion and generate macroscopic control signals to steer the populations of ground vehicles. The ground vehicles, on the other hand, will be divided into teams that are autonomously formed through learning. Each team uses the higher-layer information for tracking detailed microscopic dynamics of the target while respecting their individual local preferences and constraints.
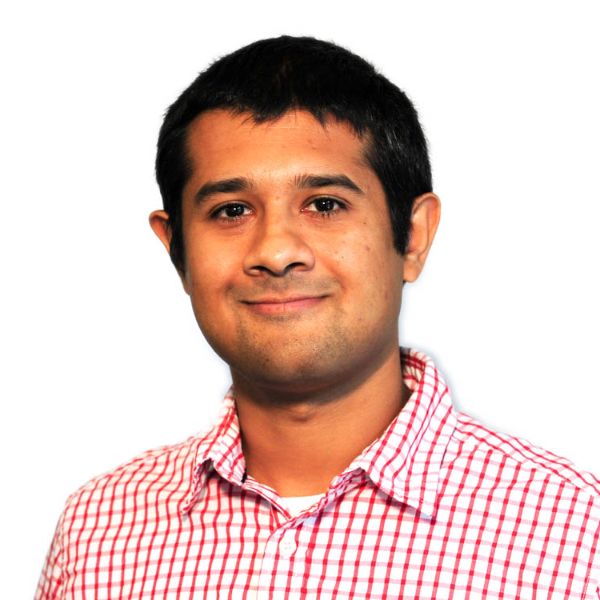
Aranya Chakrabortty, Professor, Electrical and Computer Engineering
“We strongly believe that this multi-layer, hierarchical, data-driven controller will become a seminal mechanism for the future Army’s movement, sustainment, and maneuverability,” Chakrabortty said. He and his postdoctoral researcher Ganghsan Jing are currently working with ARL and Oklahoma State to develop more online, data-driven approaches for autonomous maneuvering and sensing, not just for a single robotic agent or small-scale robotic teams, but for significantly larger-scale robotic teams. “Our methods will come in handy especially when sensing, learning, and decision-making all need to be done very fast despite the data volume being gigantic,” he said.
The paper is jointly authored by He Bai, an assistant professor in the Mechanical and Aerospace Engineering department at Oklahoma State University, Jemin George of the U.S. Army Combat Capabilities Development Command’s Army Research Laboratory, and Chakrabortty. The Army funded this effort through the Director’s Research Award for External Collaborative Initiative, a laboratory program to stimulate and support new and innovative research in collaboration with external partners. Partial funding is also provided by the US National Science Foundation (NSF) through a recent grant under its cyber-physical system (CPS) program.