Interpretable Machine Learning: Optimal Decision Trees and Optimal Scoring Systems
How do patients and doctors know that they can trust predictions from a model that they cannot understand? Transparency in machine learning models is critical in high stakes decisions, like those made every day in healthcare. My lab creates machine learning algorithms for predictive models that are interpretable to human experts. I will focus on two historical hard optimization problems whose solutions are important in practice:
(1) Optimal sparse decision trees and optimal sparse rule list models. Our algorithms are highly customized branch and bound procedures. These are an alternative to CART and other greedy decision tree methods. The solutions are globally optimal according to accuracy, regularized by the number of leaves (sparsity). This problem is NP-hard with no polynomial time approximation. I will present the first practical algorithms for this problem.
(2) Optimal scoring systems. Scoring systems are sparse linear models with integer coefficients. Traditionally, scoring systems have been designed using manual feature elimination on logistic regression models, with a post-processing step where coefficients have been rounded. However, this process does not produce optimal solutions. I will present a novel cutting plane method for producing scoring systems from data. The solutions are globally optimal according to the logistic loss, regularized by the number of terms (sparsity), with coefficients constrained to be integers.
These algorithms have been used for many medical applications and criminal justice applications.
Work with Margo Seltzer and Berk Ustun, as well as Elaine Angelino, Nicolas Larus-Stone, Daniel Alabi, Sean Hu, and Jimmy Lin.
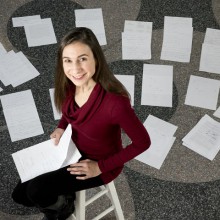
Prof. Cynthia Rudin
Professor, Duke University on September 27, 2019 at 11:45 AM in EB2 1230
Cynthia Rudin is a professor of computer science, electrical and computer engineering, and statistical science at Duke University, and directs the Prediction Analysis Lab, whose main focus is in interpretable machine learning. Previously, Prof. Rudin held positions at MIT, Columbia, and NYU. She holds an undergraduate degree from the University at Buffalo, and a Ph.D. from Princeton University. She is a three time winner of the INFORMS Innovative Applications in Analytics Award, was named as one of the “Top 40 Under 40” by Poets and Quants in 2015, and was named by Businessinsider.com as one of the 12 most impressive professors at MIT in 2015. She is past chair of both the INFORMS Data Mining Section and the Statistical Learning and Data Science section of the American Statistical Association. She has also served on committees for DARPA, the National Institute of Justice, and AAAI. She has served on three committees for the National Academies of Sciences, Engineering and Medicine, including the Committee on Applied and Theoretical Statistics, the Committee on Law and Justice, and the Committee on Analytic Research Foundations for the Next-Generation Electric Grid. She is a fellow of the American Statistical Association and a fellow of the Institute of Mathematical Statistics. She will be the Thomas Langford Lecturer at Duke University during the 2019-2020 academic year.

The Department of Electrical and Computer Engineering hosts a regularly scheduled seminar series with preeminent and leading reseachers in the US and the world, to help promote North Carolina as a center of innovation and knowledge and to ensure safeguarding its place of leading research.