Investigating the basis of noise-robust object recognition in humans and convolutional neural networks
It has been claimed that convolutional neural networks (CNNs) have now achieved human-level performance at object recognition tasks. However, modest changes to the object stimuli or to the viewing conditions can sometimes cause state-of-the-art CNNs to fail, raising questions as to whether they truly process visual information in a manner that mimics the human visual system. Here, I will present behavioral and neuroimaging data demonstrating the robustness of human vision when tasked with recognizing objects in severe levels of visual noise. Our functional MRI studies demonstrate the powerful role of top-down attentional feedback in dampening neural responses to visual noise, clutter, and competing overlapping objects. In experiments that directly pit human observers and CNNs, we find that humans outperform CNNs by a large margin and that they are affected by white noise and spatially correlated (‘pink’) noise in qualitatively different ways. We developed a noise-training procedure, generating noisy images of objects with low signal-to-noise ratio, to investigate whether CNNs can acquire robustness that better matches human vision. After noise training, CNNs could outperform human observers while exhibiting more similar qualitative patterns of performance. Moreover, noise-trained CNNs provided a better model for predicting human recognition thresholds on an image-by-image basis. Layer-specific analyses revealed that the contaminating effects of noise were dampened, rather than amplified, across successive stages of the noise-trained network. Our findings suggest that CNNs can learn noise-robust representations that better approximate human visual processing, though it remains an open question as to how the incorporation of top-down attention mechanisms might further improve the correspondence between artificial and biological visual systems.
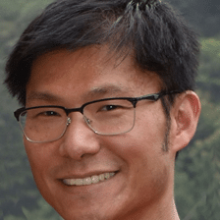
Prof. Frank Tong
Professor, Vanderbilt University on November 6, 2020 at 11:45 AM in Zoom Webinar
Join Zoom Webinar
Frank Tong is a Centennial Professor of Psychology and Professor of Ophthalmology and Visual Sciences at Vanderbilt University. He received his Ph.D. from Harvard University in 1999, began as Assistant Professor of Psychology at Princeton University in 2000, and moved to Vanderbilt University in 2004. Dr. Tong is recognized for pioneering multivariate pattern classification methods to decode feature-selective responses from the human visual cortex, and for developing novel computational approaches to characterize human behavioral and neural performance. Dr. Tong is a recipient of the Scientific American 50 Award (2005), Young Investigator Awards from the Cognitive Neuroscience Society (2006) and Vision Sciences Society (2009), and the Troland Research Award from the National Academy of Sciences (2010). In recent years, his lab has begun to explore the abilities and limitations of CNNs in challenging tasks of object recognition.

The Department of Electrical and Computer Engineering hosts a regularly scheduled seminar series with preeminent and leading reseachers in the US and the world, to help promote North Carolina as a center of innovation and knowledge and to ensure safeguarding its place of leading research.