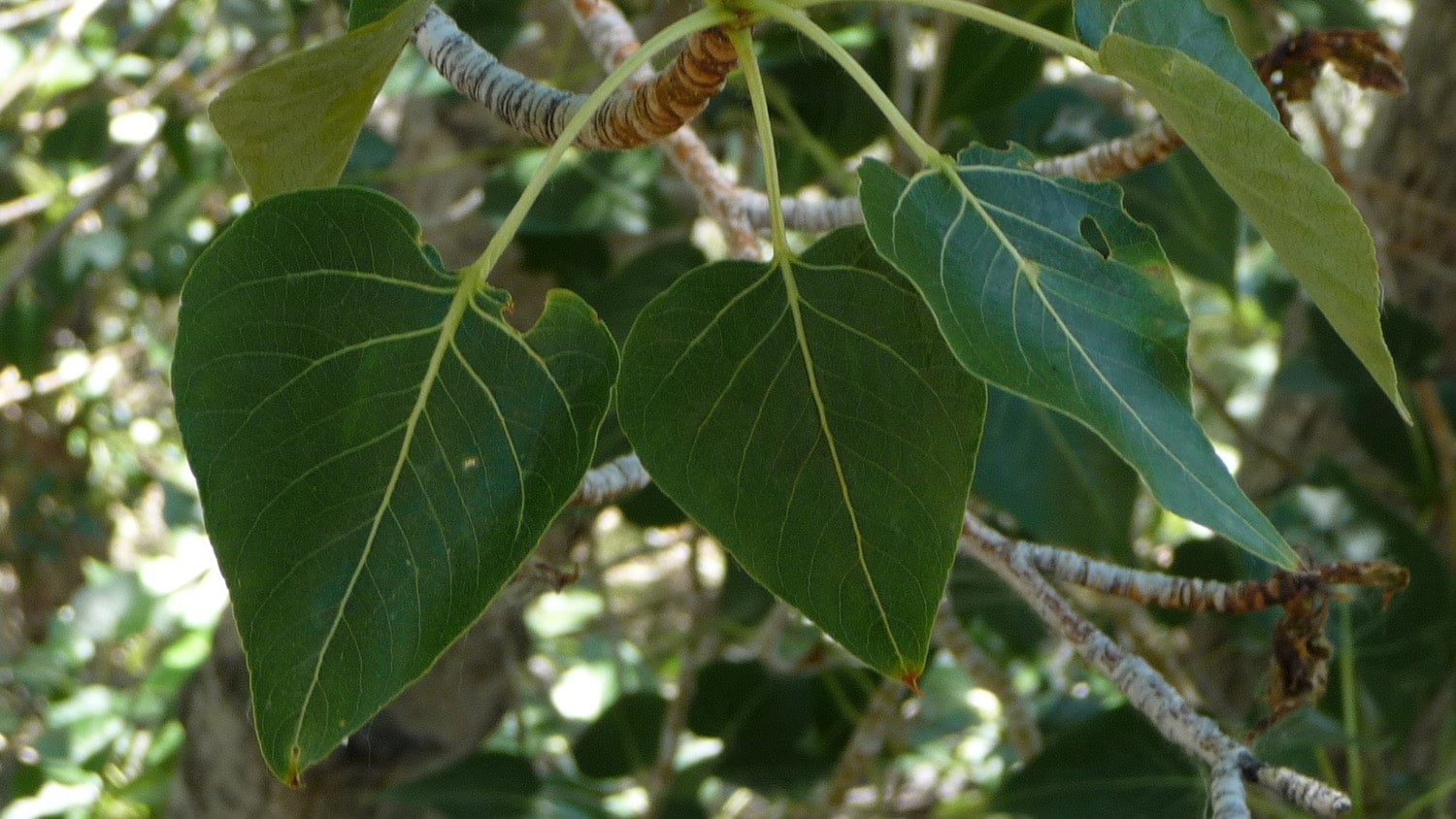
Better Predicting the Unpredictable Byproducts of Genetic Modification
How a new model may pave the way for more efficient research in the fields of genetic modification and forestry.
April 13, 2020 Staff
Researchers are interested in genetically modifying trees for a variety of applications, from biofuels to paper production. They also want to steer clear of modifications with unintended consequences. These consequences can arise when intended modifications to one gene results in unexpected changes to other genes. A new model aims to predict these changes, helping to avoid unintended consequences, and hopefully paving the way for more efficient research in the fields of genetic modification and forestry.
The research at issue focuses on lignin, a complex material found in trees that helps to give trees their structure. It is, in effect, what makes wood feel like wood.
“Whether you want to use wood as a biofuel source or to create pulp and paper products, there is a desire to modify the chemical structure of lignin by manipulating lignin-specific genes, resulting in lignin that is easier to break down,” says Cranos Williams, corresponding author of a paper on the work and an associate professor of electrical and computer engineering at NC State. “However, you don’t want to make changes to a tree’s genome that compromise its ability to grow or thrive.”
The researchers focused on a tree called Populus trichocarpa, which is a widely used model organism – meaning that scientists who study genetics and tree biology spend a lot of time studying P. trichocarpa.
“Previous research generated models that predict how independent changes to the expression of lignin genes impacted lignin characteristics,” says Megan Matthews, first author of the paper, a former Ph.D. student at NC State and a current postdoc at the University of Illinois. “These models, however, do not account for cross-regulatory influences between the genes. So, when we modify a targeted gene, the existing models do not accurately predict the changes we see in how non-targeted genes are being expressed. Not capturing these changes in expression of non-targeted genes hinders our ability to develop accurate gene-modification strategies, increasing the possibility of unintended outcomes in lignin and wood traits.
“To address this challenge, we developed a model that was able to predict the direct and indirect changes across all of the lignin genes, capturing the effects of multiple types of regulation. This allows us to predict how the expression of the non-targeted genes is impacted, as well as the expression of the targeted genes,” Matthews says.
“Another of the key merits of this work, versus other models of gene regulation, is that previous models only looked at how the RNA is impacted when genes are modified,” Matthews says. “Those models assume the proteins will be impacted in the same way, but that’s not always the case. Our model is able to capture some of the changes to proteins that aren’t seen in the RNA, or vice versa.
“This model could be incorporated into larger, multi-scale models, providing a computational tool for exploring new approaches to genetically modifying tree species to improve lignin traits for use in a variety of industry sectors.”
In other words, by changing one gene, researchers can accidentally mess things up with other genes, creating trees that aren’t what they want. The new model can help researchers figure out how to avoid that.
The paper, “Modeling cross-regulatory influences on monolignol transcripts and proteins under single and combinatorial gene knockdowns in Populus trichocarpa,” is published in the journal PLOS Computational Biology. The paper was co-authored by Ronald Sederoff, a professor emeritus of forestry and environmental resources at NC State; Jack Wang, an assistant professor of forestry and environmental resources at NC State; and Vincent Chiang, a Jordan Family Distinguished Professor Emeritus and Alumni Outstanding Research Professor with the Forest Biotechnology Group at NC State.
This work was supported by the National Science Foundation Grant DBI-0922391 to Chiang and by a National Physical Science Consortium Graduate Fellowship to Matthews.
-shipman-
Note to Editors: The study abstract follows.
“Modeling cross-regulatory influences on monolignol transcripts and proteins under single and combinatorial gene knockdowns in Populus trichocarpa”
Authors: Megan L. Matthews, Ronald Sederoff and Cranos M. Williams, North Carolina State University; Jack P. Wang and Vincent L. Chiang, Northeast Forestry University, Harbin, China, and North Carolina State University
Published: April 10, PLOS Computational Biology
Abstract: Accurate manipulation of metabolites in monolignol biosynthesis is a key step for controlling lignin content, structure, and other wood properties important to the bioenergy and biomaterial industries. A crucial component of this strategy is predicting how single and combinatorial knockdowns of monolignol specific gene transcripts influence the abundance of monolignol proteins, which are the driving mechanisms of monolignol biosynthesis. Computational models have been developed to estimate protein abundances from transcript perturbations of monolignol specific genes. The accuracy of these models, however, is hindered by their inability to capture indirect regulatory influences on other pathway genes. Here, we examine the manifestation of these indirect influences on transgenic transcript and protein abundances, identifying putative indirect regulatory influences that occur when one or more specific monolignol pathway genes are perturbed. We created a computational model using sparse maximum likelihood to estimate the resulting monolignol transcript and protein abundances in transgenic Populus trichocarpa based on targeted knockdowns of specific monolignol genes. Using in-silico simulations of this model and root mean square error, we showed that our model more accurately estimated transcript and protein abundances, in comparison to previous models, when individual and families of monolignol genes were perturbed. We leveraged insight from the inferred network structure obtained from our model to identify potential genes, including PtrHCT, PtrCAD, and Ptr4CL, involved in post-transcriptional and/or post-translational regulation. Our model provides a useful computational tool for exploring the cascaded impact of single and combinatorial modifications of monolignol specific genes on lignin and other wood properties.
Cranos Williams
Goodnight Distinguished Professor of Agricultural AnalyticsPlatform Director, NC Plant Sciences Initiative